Raymond C. Stevens
Director of The Bridge Institute,
Raymond Stevens Lab, University of Southern California
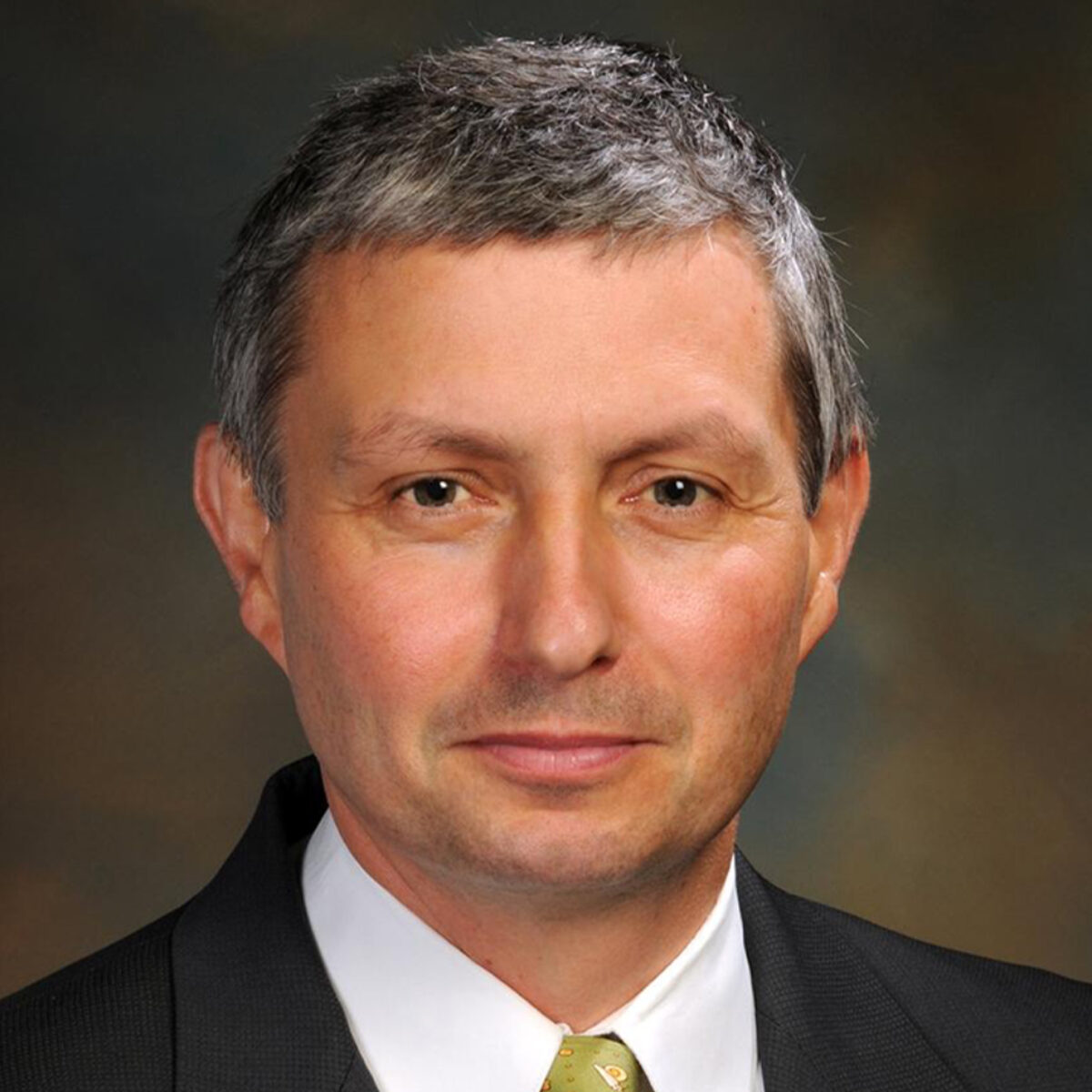
Raymond C. Stevens (born 1963) is an American chemist and structural biologist, Director of the iHuman Institute, ShanghaiTech University and Provost Professor of Biological Sciences and Chemistry, and Director of the Bridge Institute at the University of Southern California.
Stevens has started four biotechnology companies (Syrrx (1999), MemRx (2002), Receptos (2009), and RuiYi (2011)), all focused on structure based drug discovery and each company started with one of his former Ph.D. students.
Ray Stevens at the Bridge Institute leverages the most creative minds in chemistry, biology, medicine, mathematics, engineering, physics and nanosciences — as well as experts in such areas as animation and cinematography — to build the first atomic resolution structure of man.
I want to be Christopher Columbus. I like to explore. I do it both in personal life. You know, I like to travel. I like to see new places. I like to meet new people, new civilizations, new you know, new anything. And science is the same exact way. I mean, we get to see something that nobody else has ever seen before. We also get to explore, use our creativity to try to explore things that we don't know the answer to. And that's something that, being an explorer, is something that I really enjoy.
"Right now, our understanding of cells is at the same stage as the earth being flat."
Coming up with a model of a pancreatic data cell is to me a very creative thing. We really don't know you know, we have these electro micro grabbed pictures of it, of what it is, but we really don't understand how it all works together. And so its gonna take our imagination, it’s gonna take our fantasizing about what's going on inside the cell, trying to put together and understand how it works. How do these cells, as pancreatic beta cells and alpha cells, how do they control the metabiosis of our bodies.
"Scientists are not gonna solve this problem of integration of data."
I think that we were so much more creative, a long time ago. What's changed is the pace of scientific discovery. Overall I think it's largely because the pace of science is so much faster than it was in the 1960s and '70s. And even '80s. And so, what's you know, what's visionary and what can be done almost in real time now. It's just changed, we've lost a degree of perspective.You imagine something now and it becomes reality three or six months later.
We have the ability, anybody, absolutely any one of us, anybody anywhere, has the ability to change the world, and one person can change the world. That's been shown I think over and over again.
I think that trying to reduce human suffering, is also something that I sort of look up to. You know one of the things that drives me on this project, is I came to the conclusion about five years ago that we could continue developing more companies that make more drugs. We could do that, we're actually good at it. And we have a high success rate, so I think that, that's a good thing. But our diagnosis of disease is horrible. The way that we diagnose disease today, is still the same way that we diagnosed disease 100 years ago. We wait until we see symptoms. We wait until we see pain and suffering.
If you have multiple sclerosis, you don't know until you start losing the feeling in your arm, and you start having pain that's just unbearable. Cancer. You don't know that you have cancer, until you've got a good old tumor you know, growing inside you that's ultimately trying to kill you. So you know, our ability to diagnose disease is very, very old, the way that we do it. Pain and suffering is our metric. So you know one of the projects that we, and one of the reasons that I came to USC, and this is largely because of the film school. I really believe that to diagnose disease, we need to be able to monitor, evaluate, scan the human body. But we don't have the technology today. And the technology that we need is better imaging technology. Scientifically we can develop that, but we don't know how to put the, all the information together. The cinema school probably has better solutions than almost anybody else on how to integrate that data together.
It's really just the integration of the information that science is struggling with. And science's solution has been, and this is largely because of the National Institute of Health (NIH), science's solution is, just collect more data. We'll figure it out, just keep collecting data, big data, we talk about big data all the time. You know big data's not the answer. The answer is, how do you integrate the data, together. And those people don't talk to each other all that well because they're speaking different languages.
One of the things I like about science, is science ultimately corrects itself. If it's wrong, if something out there is wrong, then in the end, you don't know exactly when, but eventually science will correct itself. So you know examples are, the Earth is flat. You know, it actually was flat, it was flat for a long, long time, until somebody finally proved it's not, it's round. And you know once that was sort of, that became changed then people started evolving their attitudes. You know, the same thing I can say the same thing about our understanding of cells right now, is at the same stage as the Earth being flat.
It's really desperation. Scientists by and large, are not gonna solve this problem of integration of data. We're gonna keep collecting more and more data because, we train the next generation and that generation trains the next generation, and the way that we teach is it's all about collecting data. And less about integration. Now, the good thing is, there is you know Steve Jobs, he was largely an integrator. But again he's the exception. Elon Musk, he's an integrator. You know he takes other technologies, integrates them together. So you know there's exceptions, but until we start teaching the next generation about how to integrate data, we're gonna be stuck at this.
So art was a desperation move, to try to integrate data. And you know, I don't know whether this is actually correct or not, but somehow in my brain I kind of convinced myself that the three original big users of supercomputers, and particularly of really good graphics computers, was the military, for nuclear bomb development. Weather pattern predictions. And the film industry. And I've tried sort of digging this up, and I can't find data to support this, but I you know I think those were three of the big users of supercomputers when they first came out. You know the Cray supercomputers or the silicon graphics workstations, when they first came out.
And you know what digital arts does is, it's all about integration of data, and visualization. And I think part of what's happening with science is, it's a two dimensional world. We're used to papers that we see, we publish them. We convert all of our information into two dimensional data. Bar graphs, curves and so forth. But life is three dimensional. And so we really have to convert to a more three dimensional type of language. Something that digital arts definitely can bring to the table.
"I'm fascinated by storytelling because scientists are storytellers. That's what we do."
Will we accelerate science by more AI machine learning algorithms? Or will we simply speed up our biases? That's something that's gonna be interesting to sort of watch happen in science.
The reason why we have to have this better model of a pancreatic beta cell, is we really want to understand how all these things come together. Not just one specific, GLP1 with a specific G protein, with a specific cascade. You know it's what we refer to as the reductionist approach. The reductionist approach worked, for helping us learn science, over the past 30 years or so. But again it's hit its limitation to a degree, where we need to start combining that information together.